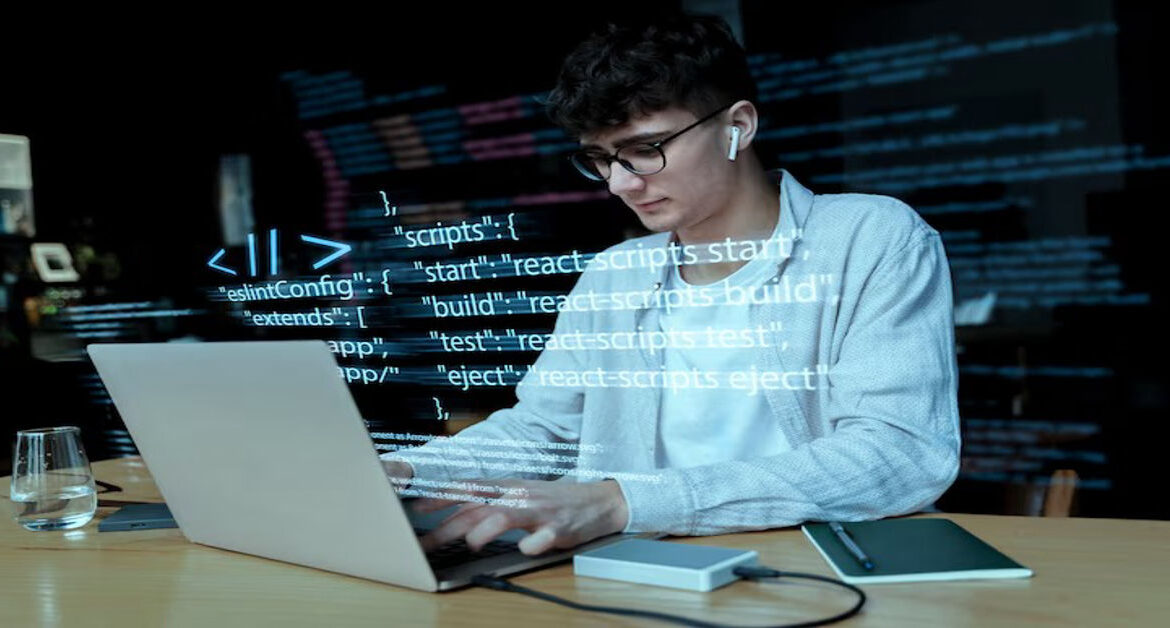
Understanding np.where in NumPy: A Complete Guide to Conditional Indexing in Python
Working with large datasets in Python requires efficient tools that can filter, transform, and analyze data with speed. One such powerful tool is np.where—a function within the NumPy library that simplifies conditional selection and indexing. Whether you’re dealing with numerical arrays or real-world data structures, understanding how np.where works can drastically streamline your code.
This article explores everything you need to know about np.where, with practical examples and insights to make your programming more effective.
What Is NumPy?
NumPy (Numerical Python) is a core library in Python that allows high-performance operations on arrays and matrices. It supports a wide range of mathematical functions and is foundational for data science, machine learning, and numerical computation.
Among its many functions, np.where stands out for its ability to apply logic-based operations directly on arrays.
Introduction to np.where
np.where is used to locate elements in an array that satisfy a particular condition. It can also return elements from one array or another depending on whether the condition is true or false.
Think of it as the NumPy equivalent of an if-else statement that works on entire arrays without looping.
Syntax and Parameters
numpy.where(condition, [x, y])
- condition: A boolean array or expression that determines which elements to select.
- x: Optional. Values to return where the condition is true.
- y: Optional. Values to return where the condition is false.
The function behaves differently depending on whether x and y are included.
Return Values Explained
- If only the condition is provided, np.where returns the indices where the condition is true.
- If both x and y are provided, the function returns an array of values from x where the condition is true, and from y where it is false.
This dual functionality makes np.where versatile for both indexing and conditional value selection.
Table: Quick Overview of np.where Behaviors
Case | Parameters Used | Return Value Description |
Condition only | condition | Indices where condition is True |
Condition with x and y | condition, x, y | Values from x (True) and y (False) |
Mixed type input | condition, scalar | Returns scalar values conditionally |
Basic Examples of np.where
import numpy as np
arr = np.array([1, 2, 3, 4, 5])
indices = np.where(arr > 3)
print(indices) # Output: (array([3, 4]),)
In this example, np.where returns the indices where values in the array are greater than 3.
Using np.where Without x and y
This form is used for finding positions of specific elements:
arr = np.array([[1, 2], [3, 4]])
pos = np.where(arr == 3)
print(pos) # Output: (array([1]), array([0]))
You get a tuple of arrays indicating the row and column index of the matched value.
Using np.where With x and y
You can return values based on condition using x and y:
arr = np.array([10, 20, 30, 40])
result = np.where(arr > 25, ‘High’, ‘Low’)
print(result) # Output: [‘Low’ ‘Low’ ‘High’ ‘High’]
This acts like a vectorized if-else statement, replacing loops with clean, efficient code.
Preserving Array Dimensions
If you want to preserve the original array’s shape and not just get indices, use np.where in a conditional assignment.
arr = np.array([[1, 2, 3], [4, 5, 6]])
new_arr = np.where(arr > 3, arr, -1)
print(new_arr)
# Output:
# [[-1 -1 -1]
# [ 4 5 6]]
Here, you keep the array structure intact while modifying only selected values.
Real-World Applications
- Data Cleaning: Replacing missing values or invalid entries
- Data Filtering: Selecting rows in datasets based on column values
- Image Processing: Applying conditions to pixel arrays
- Financial Analysis: Assigning risk labels based on thresholds
The use cases are limitless, especially in data-heavy tasks.
Tips for Using np.where Effectively
- Avoid overly complex conditions—break them into simpler expressions.
- Use broadcasting for multidimensional arrays.
- For performance-critical code, combine np.where with vectorized functions.
- When working with pandas, np.where can simplify conditional column creation.
Common Mistakes to Avoid
- Confusing np.where’s indexing with slicing
- Passing lists instead of arrays
- Expecting scalar outputs when arrays are returned
- Misusing np.where for operations better handled with masking
Understanding its behavior helps prevent logical errors in your scripts.
Comparing np.where With Other NumPy Methods
Method | Use Case | Output Type |
np.where | Conditional value or index selection | Indices or new array |
np.select | Multiple conditions | New array |
np.nonzero | Indices of non-zero elements | Indices |
Boolean Masking | Filter elements based on condition | Subset array |
While np.where is powerful, sometimes simpler masking or other functions are more suitable depending on the task.
Conclusion
The np.where function is one of NumPy’s most powerful tools for conditional logic. It bridges the gap between if-else control flow and efficient, readable array operations. Whether you’re trying to extract indices or build new arrays based on conditions, np.where is your go-to function.
With practical examples and a clear understanding of syntax, you can confidently use np.wher’e in your Python projects, saving time and improving code efficiency.
FAQs
What does np.wher’e return?
If only a condition is provided, it returns indices where the condition is true. If x and y are also provided, it returns an array selecting values from x and y based on the condition.
Can np.wher‘e be used with multidimensional arrays?
Yes, and it returns a tuple of arrays representing the indices across each dimension.
How is np.wher’e different from boolean indexing?
np.wher’e returns the indices or values conditionally, while boolean indexing filters an array using True/False masks.
Is np.whe’re efficient for large datasets?
Yes, it’s optimized for performance and preferred over Python loops for large arrays.
Can I use np.wher’e in pandas?
Yes, np.whe’re is often used inside pandas apply or directly to create new DataFrame columns conditionally.
Meta Description: Learn how to use np.wher’e in NumPy to perform conditional indexing and element selection. Includes examples, syntax breakdown, and real-world applications.
Archives
Calendar
M | T | W | T | F | S | S |
---|---|---|---|---|---|---|
1 | 2 | 3 | 4 | 5 | 6 | |
7 | 8 | 9 | 10 | 11 | 12 | 13 |
14 | 15 | 16 | 17 | 18 | 19 | 20 |
21 | 22 | 23 | 24 | 25 | 26 | 27 |
28 | 29 | 30 | 31 |
Leave a Reply